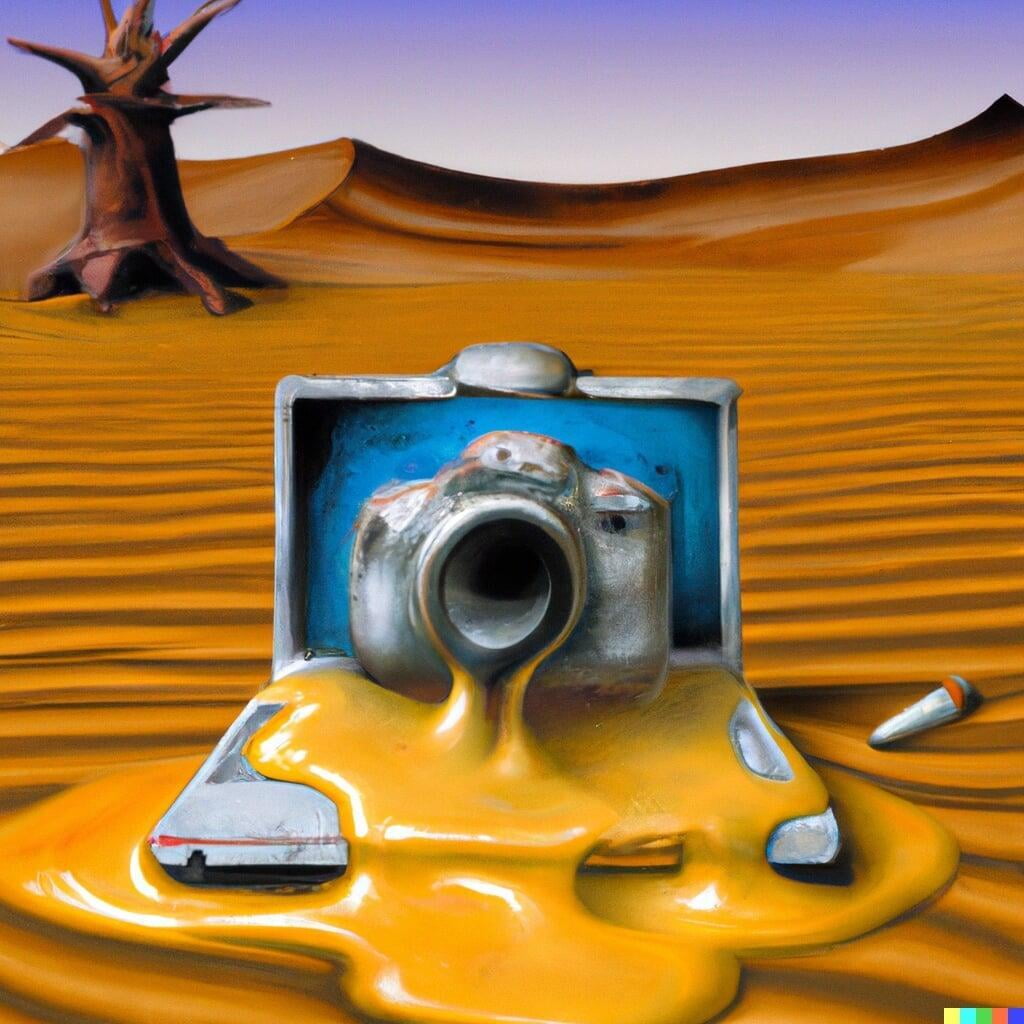
Digital HUB is an open online community of financial and data science professionals pursuing practical applications of AI in their everyday functions. Digital HUB community provides expert, curated insights into financial applications of Generative AI, Large Language Models, Machine Learning, Data Science, Crypto Assets and Blockchain.
A key focus for The Digital HUB publication is to provide best practices for the safe deployment of AI at scale such as: assessing the ability to execute, determining an organization’s digital DNA, fostering skill development, and encouraging responsible AI.
Digital HUB is an open online community of financial and data science professionals pursuing practical applications of AI in their everyday functions. Digital HUB community provides expert, curated insights into financial applications of Generative AI, Large Language Models, Machine Learning, Data Science, Crypto Assets and Blockchain.
A key focus for The Digital HUB publication is to provide best practices for the safe deployment of AI at scale such as: assessing the ability to execute, determining an organization’s digital DNA, fostering skill development, and encouraging responsible AI.
By Don Hejna, CFA, CAIA, FDP 2023-02-09
By Don Hejna, CFA, CAIA, FDP 2023-02-09
In the days before digital cameras, taking a photo was something you thought about because there were costs associated with it: film, developing, fast developing if you needed it right away, and perhaps the hiring of a professional when the quality and timeliness of the photo absolutely mattered.
Digital cameras drove down the cost of photography to near zero. As a result, millions of people began taking and sharing high quality photos. Furthermore, the technology to calculate image exposure and focus did automatically for free what many human photographers had previously spent their careers mastering.
Large language models (LLMs) promise to similarly empower the masses and disrupt industries and professions.
The era of film photography has all but ended and with it a whole industry of developing and printing images has disappeared and been replaced by one of storing, sharing, and sorting images. The need for professional photographers has dropped considerably, though for special important events you’ll still hire one, or at least a friend that’s “pretty good” at it.
Large Language Models (LLMs) will do for text generation and knowledge retrieval what the digital camera did for film-based photography. You’ll still hire a professional now and then, but as with photos, the difference in results from a computer and professional will narrow and for many common information tasks, the difference in price for similar results just won’t justify the professional’s cost.
This change in how knowledge is generated and organized will be even more disruptive and empowering to more industries because it will touch on so many aspects of knowledge and creativity: text information, knowledge, domain specialization, planning, art, business, investing, chat-bots, and question and answer dialogues.
Even more exciting is the fact that we don’t know where the progress will level off. Consider: Many of the innovations in AI brought about by LLMs were actually complete surprises to the creators of those models. More on this in a bit.
HOW DID LLMS GET SO SMART? --A FUNNY THING HAPPENED ON THE WAY TO THE SHOW…
How did LLMs get so smart? What started as a way of modeling language (Natural Language Processing, NLP for short) emerged as a way of modeling knowledge at a level that surprised everyone, including the designers themselves at times. Early models were focused on translating between two languages using an architecture dubbed a “transformer”. Transformers took one kind of input, for example a page of English text, and a desired output, for example the same page content in French, and optimized neural networks in the transformer to create trained models that could produce the correct translation in a step-by-step manner. Interestingly enough, the trained models could also output or generate realistic natural and coherent text: first fragments and phrases, and then quickly, full paragraphs and pages.
What LLMs are really doing is compressing, associating, and storing representations of strings of words (language). Since most of the language samples used in training were written by humans and intended to convey knowledge, the compressed, associated, and stored representations of these word strings are in essence stored knowledge. The ability of LLMs, and transformers in particular, to train on unlabeled existing text is critical because it meant the models could basically “learn” by reading existing text with a few simple and automated perturbations, such as “masking” words and predicting the next word in a series.
The “happy accident” of lots of data, cheap computation (GPU’s), and large memory models, resulted in the ability to compress and associate data into “latent” (hidden) states. Those latent states or more accurately the representation of them in a computer, when tickled with similar (nearby) data, as a prompt, tend to laugh in the local language of that data. This is to say, if after compressing the information of SEC filings to a region of memory in a LLM model, if one asks about risks and GAAP accounting, the model will begin to recite intelligent prose on that subject that can go on for as long or as short as requested. Much like the way a tickle in one’s throat causes a coughing fit, or a well-timed joke can lead to someone spraying a mouthful of their soda on their dinner companions, asking a question leads to surprising results.
While working hard to solve one problem (NLP) the systems began to display an uncanny ability to compress and associate data of various types. Using language as a proxy for understanding and knowledge, the ability to associate knowledge with snippets of text in the form of questions, or create art based on short word phrases appears outright intelligent.
EMERGENCE-Y: WELCOMED AND UNEXPECTED SURPRISES IN ABILITIES
Researchers noted that larger LLMs trained on larger datasets tended to perform much better than smaller ones trained with less data; and as researchers pushed the limits of model size and training sets for better performance, several interesting and unexpected abilities emerged. These behaviors are dubbed “emergent” and are, by definition, unpredictable surprises that appear to be enabled by sheer size alone.
An ability is emergent if it is not present in smaller models but is present in larger models. i
What this means is that as an LLM model’s size (number of parameters) grows, it often gains the ability to perform a task that was not possible using a smaller version of the same LLM architecture. It’s as if by magic, the model turns a corner and gains an ability to produce a high quality result. How this happens is an area of active research.
Some examples of emergent abilities in LLMs include the ability to perform addition and subtraction, and even multiplication; the ability to generate truthful answers; and the ability to answer knowledge based questions across a large domain of topics.
A DURABLE AND FLEXIBLE ARCHITECTURE
Emergent behavior should not be confused with architecture changes that accomplish new tasks. The core “transformer” architecture has remained durable and useful across many domains as innovators find ways to reuse this successful serialization and transformer approach.
After utilizing the transformer architecture for text, some very clever researchers applied the technique to images by “serializing” an image into subblocks in a way that allowed the image to be represented as a sequence. (Think scan lines on older interlaced televisions, but with small blocks of a digital image.) Then using a transformer architecture to “learn” the association of text with a serialized image, an LLM dubbed “Dall-E” was born. Thus, images could now be generated from a few keywords. Clever readers may be wondering how a scanned image is recreated and the answer is roughly that as long as the serialization during training is consistent across all images, the model learns how to build 2D pictures from the subblocks even though vertical blocks are disjointed in the serialization.
The Dall-E model used a novel scanning technique to turn images into sequences of image fragments that could then be associated with text (often just image captions). The transformer architecture “learned” how to parse and associate serialized blocks of images with text and surprisingly, how to generate images in the reverse manner: take text and input and serialize blocks of images that when reassembled formed coherent images.
The ability to generate images was surprising, yet even more surprising was the model’s ability to make a linedrawing sketch version of a picture by asking the model to make “the exact same cat on the top as a sketch at the bottom”.ii
It was like asking the newly hired novice barista for a latte with milk and receiving an image of Picasso’s Guernica created in the foam. You’d be right to ask, “How on earth did you learn this?” And the answer would be, “I learned by watching the professionals and mimicking how they did it.”
The latest models are taking disparate representations of sequential data and learning to associate, compress, and represent them and combine them in ways that allow a computer to create an image of an aardvark riding a tricycle. And in many cases the results have been surprisingly high quality.
The latest break-through models utilizing transformers incorporate a so called “generalist agent”iii to operate on a wide variety of inputs allowing LLMs (or their equivalents) to expand their learning far beyond text to such areas as playing Atari games, stacking blocks with a robotic arm, describing images and creating images or captions.
WHAT TRANSFORMER TECHNOLOGIES WILL CONTINUE TO DISRUPT
Back to the camera analogy: Pre “Dall-E” if I needed an artistic rendering of an aardvark on a tricycle, I would hire a graphic artist to create one. It would take a few hours for them to create an image or a few variants, and depending on their patience and my budget, I’d have a few renderings to choose from after a few days.
Today, transformers can provide hundreds of variants in seconds at near zero cost. More sophisticated models can produce images and make incremental changes such as changing the color of the car from blue to red, removing the need for photo editing software and the skilled graphic artist to run it.
In legal fields, contracts and policies can be generated automatically, and although one would be foolhardy to use such a document today without proofreading it, it remains much easier and faster to correct errors than generate whole documents from scratch. Indeed, if you ask ChatGPT to develop a privacy policy for your website, you’ll get a very good prototype based on the aggregated data of thousands of policies used during the model’s training.
Text to speech, speech to text, text to images, and question and answer dialogues for things like customer support and searching will all be disrupted and likely widely deployed and adopted at near zero costs as more and more LLMs are developed and deployed.
ECONOMICS AND WHERE THE DISRUPTION WILL TAKE PLACE:
The short answer is anywhere you can serialize a sequence of events or information and present a serialized desired output stream with a score, transformers have the ability to outperform humans given enough data. For large segments of the economy LLMs will provide alternatives to a live person that are “good enough” that you don’t need a human.
Today we see ChatGPT working with text and Dall-E working with images, but the future is already expanding to tasks and procedures that can be serialized as well. “Make me an omelet with only egg-whites.” will be next.
This means the often human-centered job of talking to a customer, sorting through facts to determine what’s needed and then supplying information to solve a problem or place an order, will likely be handled by LLMs.
The human task of generating content can now be performed by LLMs cheaply and quickly, producing results that are more exhaustive and grammatically correct at the outset. The human task of becoming an expert in a field such as customer support, while extremely valuable, is also likely to be mimicked easily by a LLM that is trained on a large volume of output from such experts. One can’t help but wonder, where does that leave the experts in the era of LLMs? In finance the traditional bespoke investment advice model relies on a well understood set of facts and rules being customized to a particular individual or entity’s situation: the amount of assets, the type of assets, risk tolerance, investment horizon, and a schedule of desired cash flows. This situational data (the input) is then provided as input along with statistical metrics for a “universe” of investable assets to develop an allocation (the output). All of these inputs and outputs are easily serialized meaning the ability to study and intelligently mimic investment situations is not far off once a model has access to many recommendations. For billion-dollar investments, you’ll likely want a human making decisions, but for the majority of investors, LLMs are likely to do well enough.
In summary, we’ll still need experts, just far fewer of them. Very much like professional photographers after the emergence of digital cameras: For very important things you’ll hire human experts, but for most other things, the LLMs will perform at the level required for fractions of the price, or free.
LIMITS OF LLMS
It is important to keep in mind that current LLMs gain all their knowledge from training sets of data that exist in the past. For many forms of information this is adequate. However, for situations that are newly emerging, such as a pandemic, unless retrained, LLMs will be of little use since they cannot answer questions about data they have not previously encountered.
CLOSING THOUGHTS
Before worrying that a new era of human obsolescence is upon us, consider the benefits of digital photography. Most of society would agree that the world in which film based photography is obsolete is better: inexpensive high quality photos have benefited science and humanity (save only perhaps those photographers and companies dedicated to the film-based medium). In reality, some film-based photographers are now sought after for the rarified aspects of film photography. But alas, there are fewer of them despite there being more images than ever. If the future rhymes with the past in this respect, most of society will benefit from information and knowledge provided by LLMs even as the demand for some experts drops and their numbers decline. To me, society doing more “expert things” with less experts feels like progress.
PS. None of this article was written by an LLM.
iEmergent Abilities of Large Language Models, https://doi.org/10.48550/arXiv.2206.07682
iiZero-Shot Text-to-Image Generation, https://doi.org/10.48550/arXiv.2102.12092
iii A Generalist Agent, https://doi.org/10.48550/arXiv.2205.06175