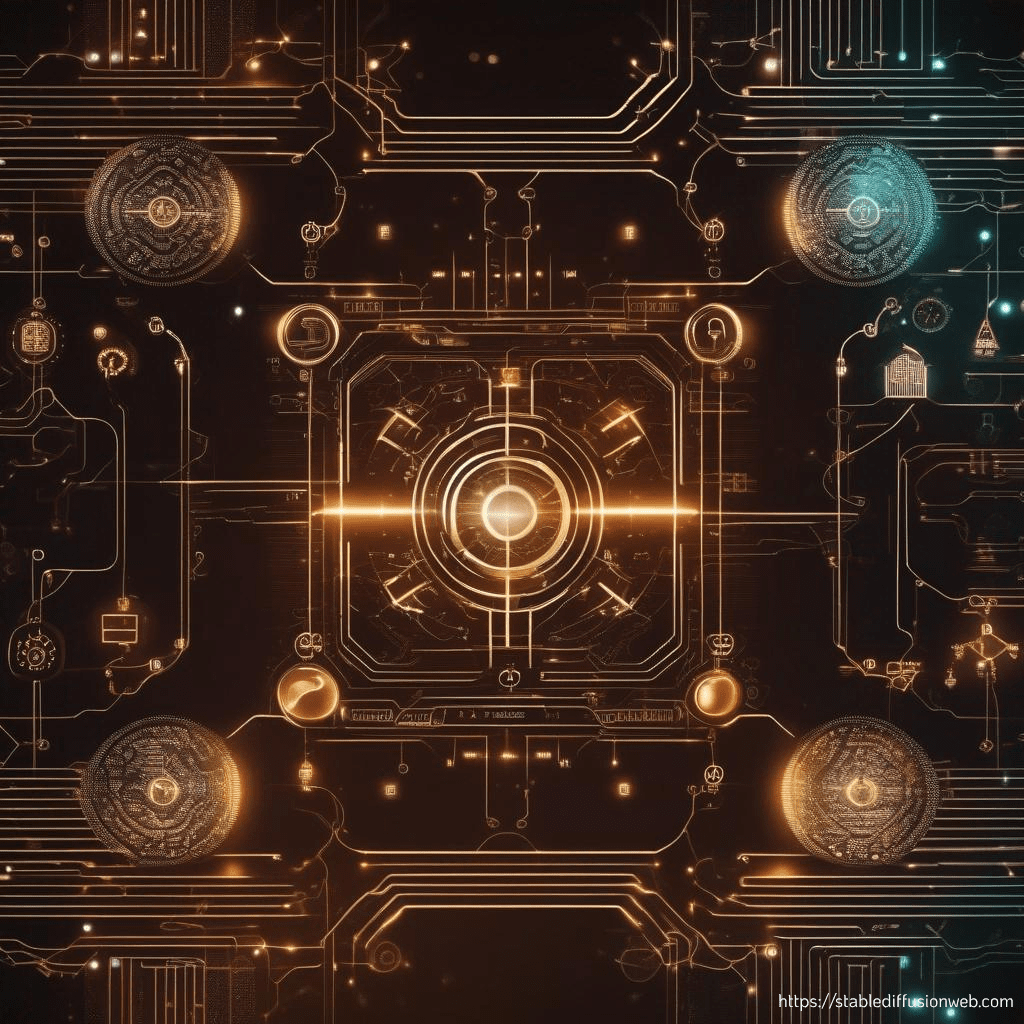
Digital HUB is an open online community of financial and data science professionals pursuing practical applications of AI in their everyday functions. Digital HUB community provides expert, curated insights into financial applications of Generative AI, Large Language Models, Machine Learning, Data Science, Crypto Assets and Blockchain.
A key focus for The Digital HUB publication is to provide best practices for the safe deployment of AI at scale such as: assessing the ability to execute, determining an organization’s digital DNA, fostering skill development, and encouraging responsible AI.
Digital HUB is an open online community of financial and data science professionals pursuing practical applications of AI in their everyday functions. Digital HUB community provides expert, curated insights into financial applications of Generative AI, Large Language Models, Machine Learning, Data Science, Crypto Assets and Blockchain.
A key focus for The Digital HUB publication is to provide best practices for the safe deployment of AI at scale such as: assessing the ability to execute, determining an organization’s digital DNA, fostering skill development, and encouraging responsible AI.
The race to larger Large Language Models (LLMs) has reached a new high. The generative AI models sizes, measured by the number of parameters, have grown 5000X in the last five years! The latest OpenAI GPT-4 is rumored to be a mixture of 8 x 220-billion-parameter = 1.7 Trillion parameters! These incredible innovations around generative AI, were achieved by a handful of labs around the world some of which are shown in this graph. They have shown that these model architectures can be scaled and have achieved remarkable results in many fields including medicine, chemistry, drug discovery, legal, finance, software development, chip design, and general knowledge. These achievements come with costs including huge compute power, massive data sets, and lots of human feedback.
So, where do we go from here? There are several trends emerging: 1) Larger models: continue with larger models (or mixture of models) that can only be served through Cloud applications (like ChatGPT) or major data centers. This direction will put the power and innovation in the hands of few organizations that can afford the high costs and better control misuse; 2) Open-Source VS Closed: current trend in proprietary models VS open-source would further concentrate the development and innovation in the hands of a few. I have created a graphic visualizing this trend here. Open-source models serve many purposes including scientific audit, enable startups, and increase accessibility by many; 3) Smaller and more specialized models: this is an alternative strategy to the current “bigger is better” trend. Instead of focusing on scale and size, development labs can train smaller, more efficient models and make them accessible to the larger AI community to be fine-tuned for applications without having to train models from scratch. More on this trend here. This option is best suited for variety of enterprises where compute power is limited, and proprietary business operations data is required in addition to the pre-training data – you can’t run a manufacturing floor on the internet data!
With these trends in mind, the main take aways are: 1) pace of technology development is much faster than the pace of adoption by industries. Real challenge is to convert these innovations to the real-world everyday operation of businesses; 2) safety, security, and operational reliability of AI models is paramount in deployment in the industries. The smaller more efficient models will allow better control by the businesses that are responsible for their outcomes—developing features like watermarks for machine-generated content, more reliable safety filters, and the ability to cite sources when generating answers to questions—can also contribute toward making LLMs more accessible and robust; 3) classical machine learning models are still very efficient and inexpensive for industrial applications. Current excitement around generative AI tend to take most of the executive’s attention to the detriment of machine learning techniques.
The race to larger Large Language Models (LLMs) has reached a new high. The generative AI models sizes, measured by the number of parameters, have grown 5000X in the last five years! The latest OpenAI GPT-4 is rumored to be a mixture of 8 x 220-billion-parameter = 1.7 Trillion parameters! These incredible innovations around generative AI, were achieved by a handful of labs around the world some of which are shown in this graph. They have shown that these model architectures can be scaled and have achieved remarkable results in many fields including medicine, chemistry, drug discovery, legal, finance, software development, chip design, and general knowledge. These achievements come with costs including huge compute power, massive data sets, and lots of human feedback.
So, where do we go from here? There are several trends emerging: 1) Larger models: continue with larger models (or mixture of models) that can only be served through Cloud applications (like ChatGPT) or major data centers. This direction will put the power and innovation in the hands of few organizations that can afford the high costs and better control misuse; 2) Open-Source VS Closed: current trend in proprietary models VS open-source would further concentrate the development and innovation in the hands of a few. I have created a graphic visualizing this trend here. Open-source models serve many purposes including scientific audit, enable startups, and increase accessibility by many; 3) Smaller and more specialized models: this is an alternative strategy to the current “bigger is better” trend. Instead of focusing on scale and size, development labs can train smaller, more efficient models and make them accessible to the larger AI community to be fine-tuned for applications without having to train models from scratch. More on this trend here. This option is best suited for variety of enterprises where compute power is limited, and proprietary business operations data is required in addition to the pre-training data – you can’t run a manufacturing floor on the internet data!
With these trends in mind, the main take aways are: 1) pace of technology development is much faster than the pace of adoption by industries. Real challenge is to convert these innovations to the real-world everyday operation of businesses; 2) safety, security, and operational reliability of AI models is paramount in deployment in the industries. The smaller more efficient models will allow better control by the businesses that are responsible for their outcomes—developing features like watermarks for machine-generated content, more reliable safety filters, and the ability to cite sources when generating answers to questions—can also contribute toward making LLMs more accessible and robust; 3) classical machine learning models are still very efficient and inexpensive for industrial applications. Current excitement around generative AI tend to take most of the executive’s attention to the detriment of machine learning techniques.
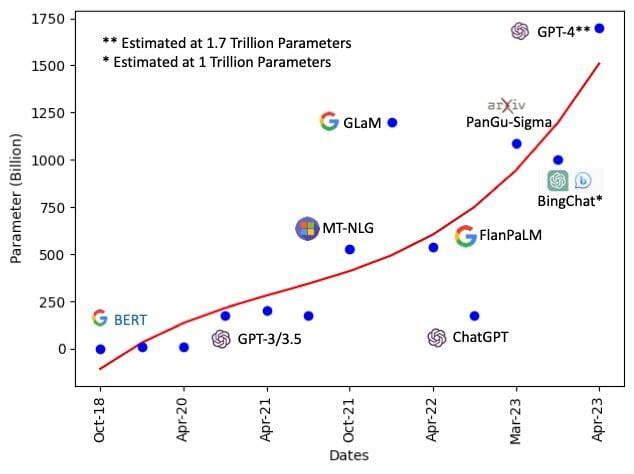